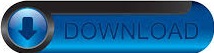
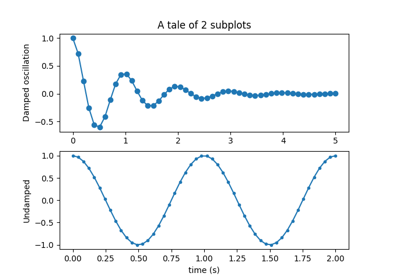
This code zs = _matrix()įig, axs = plt.subplots(tight_layout=True)Īxs. However, if I plot the histogram on its own, the problem disappears. The figure produced is the following (sorry, I don't have the reputation to put the image inline):Īs you can see the histogram subplot is empty. conda_env/lib/python3.7/site-packages/numpy/lib/histograms.py:825: RuntimeWarning: invalid value encountered in less_equal Example 1 : Python3 import matplotlib. Produces the following output /conda_env/lib/python3.7/site-packages/numpy/lib/histograms.py:824: RuntimeWarning: invalid value encountered in greater_equal keep = (tmp_a >= first_edge) Method 1 : We can pass an integer in bins stating how many bins/towers to be created in the histogram and the width of each bin is then changed accordingly. from Weights', ylabel='Exact Population', title='Weight Check')Īxs.ticklabel_format(useOffset=False, style='plain')Īxs.set(xlabel='Relative Error', ylabel='Frequency') Model = LinearRegression(fit_intercept = False).fit(X,y)įig, axs = plt.subplots(2, 1, tight_layout=True)Īxs.set(xlabel='Pop. This is the part of the code creating the plot - CZs is a Pandas DataFrame whereas LinearRegression() is imported from sklearn.linear_model: X= _matrix() I am trying to plot a histogram as a subplot below a scatterplot. It is always advisable to check that your impressions of the distribution are consistent across different bin sizes.I am new to matplotlib. But you should not be over-reliant on such automatic approaches, because they depend on particular assumptions about the structure of your data. By default, displot()/ histplot() choose a default bin size based on the variance of the data and the number of observations. Parameters dataDataFrame The pandas object holding the data.
#Pyplot subplot histogram series#
This function calls (), on each series in the DataFrame, resulting in one histogram per column. A histogram is a representation of the distribution of data. The size of the bins is an important parameter, and using the wrong bin size can mislead by obscuring important features of the data or by creating apparent features out of random variability. Make a histogram of the DataFrame’s columns. For instance, we can see that the most common flipper length is about 195 mm, but the distribution appears bimodal, so this one number does not represent the data well. This plot immediately affords a few insights about the flipper_length_mm variable. displot ( penguins, x = "flipper_length_mm" ) A histogram is a bar plot where the axis representing the data variable is divided into a set of discrete bins and the count of observations falling within each bin is shown using the height of the corresponding bar: This is the default approach in displot(), which uses the same underlying code as histplot(). () function itself provides many attributes with the help of which we can modify a histogram.

Perhaps the most common approach to visualizing a distribution is the histogram. It is important to understand these factors so that you can choose the best approach for your particular aim. There are several different approaches to visualizing a distribution, and each has its relative advantages and drawbacks. They are grouped together within the figure-level displot(), jointplot(), and pairplot() functions. The axes-level functions are histplot(), kdeplot(), ecdfplot(), and rugplot(). The distributions module contains several functions designed to answer questions such as these. What range do the observations cover? What is their central tendency? Are they heavily skewed in one direction? Is there evidence for bimodality? Are there significant outliers? Do the answers to these questions vary across subsets defined by other variables? Techniques for distribution visualization can provide quick answers to many important questions. In this article, we explore practical techniques that are extremely useful in your initial data analysis and plotting. An early step in any effort to analyze or model data should be to understand how the variables are distributed. Matplotlib histogram is used to visualize the frequency distribution of numeric array by splitting it to small equal-sized bins.
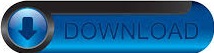